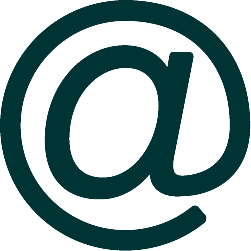
Moritz Schauer
Moritz Schauer
Associate Professor (Universitetslektor)
Department of Mathematical Sciences
Chalmers University of Technology and University of Gothenburg
Contact/E-mail
Office: Chalmers Tvärgata 3, Room H3029, 41296 Göteborg (map)
Phone: +46 31 772 3029
Mail: smoritz@chalmers.se
Github: @mschauer
University of Gothenburg Address Book / Staff Page Mathematical Sciences
Stations
2019 – | Department of Mathematical Sciences, Chalmers University of Technology and University of Gothenburg.
|
||||||||||
2015 – 2019 | Postdoc at the Mathematical Institute, University of Leiden. Projects: Causal Discovery from High-Dimensional Data in the Large-Sample Limit. | ||||||||||
2014 – 2015 | Postdoc at the Korteweg-de Vries Institute for Mathematics, University of Amsterdam, VICI project Foundations of nonparametric Bayes procedures. | ||||||||||
2010 – 2014 | PhD candidate at the Delft Institute of Applied Mathematics, Delft University of Technology in cooperation with EURANDOM and support by the STAR cluster of the Dutch Science Foundation NWO. | ||||||||||
2004 – 2009 | Diplom-Mathematik at University of Hamburg, Department Mathematical Statistics and Stochastic Processes. |
Research interest
Nonparametric Bayesian inference for diffusion processes.
Boundary value problems for S(P)DEs and conditional diffusion processes.
Bayesian inference on graphs and causal inference.
Projects
PhD project: Stochastic Continuous-Depth Neural Networks. Chalmers AI Research Centre (CHAIR), 2020-2025.
Project Exploring and Statistically Learning an Excitable Stochastic-Dynamical Model. Scholarship for Explorable Research, https://nextjournal.com, 2019-2020.
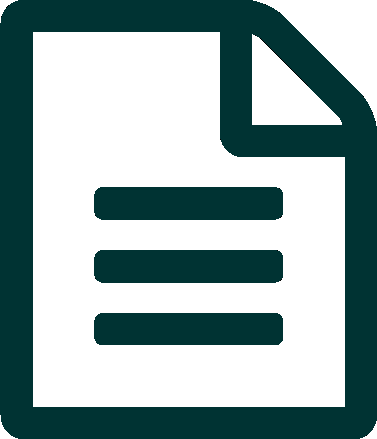
Publications
Preprints
Elizabeth L. Baker, Moritz Schauer, Stefan Sommer: Score matching for bridges without time-reversals. arXiv.2407.15455, 2024.
Gaurav Arya, Moritz Schauer, Ruben Seyer: Gradient Estimation via Differentiable Metropolis-Hastings. arXiv:2406.14451, 2024.
Marc Corstanje, Frank van der Meulen, Moritz Schauer, Stefan Sommer: Simulating conditioned diffusions on manifolds. arXiv.2403.05409, 2024.
Joris Bierkens, Sebastiano Grazzi, Gareth Roberts, Moritz Schauer: Methods and applications of PDMP samplers with boundary conditions. arXiv:2303.08023, 2023.
Frank van der Meulen, Moritz Schauer: Automatic Backward Filtering Forward Guiding for Markov processes and graphical models. arXiv:2010.03509, 2020.
Richard C. Kraaij, Moritz Schauer: A generator approach to stochastic monotonicity and propagation of order. arxiv:1804.10222, 2018.
Monography/Thesis
Moritz Schauer: Bayesian inference for discretely observed diffusion processes. Ph.D. Thesis. Delft University of Technology, 2015.
Articles
2024
Moritz Schauer, Marcel Wienöbst: Causal structure learning with momentum: Sampling distributions over Markov Equivalence Classes. Proceedings of The 12th International Conference on Probabilistic Graphical Models, PMLR 246:382-400, 2024.
2023
Gaurav Arya, Ruben Seyer, Frank Schäfer, Kartik Chandra, Alexander K. Lew, Mathieu Huot, Vikash K. Mansinghka, Jonathan Ragan-Kelley, Christopher Rackauckas, Moritz Schauer: Differentiating Metropolis-Hastings to Optimize Intractable Densities Differentiable Almost Everything Workshop of the 40th International Conference on Machine Learning, Honolulu, Hawaii, USA, arXiv:2306.07961, 2023.
Denis Belomestny, Shota Gugushvili, Moritz Schauer, Peter Spreij: Weak solutions to gamma-driven stochastic differential equations. Indagationes Mathematicae 34 (4), 820-829, 10.1016/j.indag.2023.03.004 (arXiv:2108.11891), 2023.
Frank van der Meulen, Shota Gugushvili, Moritz Schauer, Peter Spreij: Nonparametric Bayesian volatility learning under microstructure noise. Japanese Journal of Statistics and Data Science 6, pp. 551-571, 2023. 10.1007/s42081-022-00185-9 (arxiv:1805.05606).
Marc Corstanje, Frank van der Meulen, Moritz Schauer: Conditioning continuous-time Markov processes by guiding Stochastics 95 (6), 963--996, 2023. 10.1080/17442508.2022.2150081 (arXiv:2111.11377).
Joris Bierkens, Sebastiano Grazzi, Frank van der Meulen, Moritz Schauer: Sticky PDMP samplers for sparse and local inference problems. Statistics and Computing 33 (1), 2023. 10.1007/s11222-022-10180-5 (arXiv:2103.08478)
2022
Gaurav Arya, Moritz Schauer, Frank Schäfer, Chris Rackauckas: Automatic Differentiation of Programs with Discrete Randomness. Advances in Neural Information Processing Systems 35, 10435--10447, 2022.(Link, arXiv:2210.08572).
Alexis Arnaudon, Frank van der Meulen, Moritz Schauer, Stefan Sommer: Diffusion bridges for stochastic Hamiltonian systems with applications to shape analysis. SIAM Journal on Imaging Sciences 15:1, pp. 293–323, 2022. 10.1137/21M1406283 (arxiv:2002.00885).
Chad Scherrer, Moritz Schauer: Applied measure theory for probabilistic modeling. JuliaCon Proceedings, 1(1), 92, 2022. 10.21105/jcon.00092.
Raphael Sonabend, Florian Pfisterer, Alan Mishler, Moritz Schauer, Sumantrak Mukherjee, Lukas Burk and Sebastian Vollmer: Flexible group fairness metrics for survival analysis. DSHealth 2022 (Workshop on Applied Data Science for Healthcare), 2022. (arxiv:2206.03256).
Denis Belomestny, Shota Gugushvili, Moritz Schauer, Peter Spreij: Nonparametric Bayesian volatility estimation for gamma-driven stochastic differential equations. Bernoulli 28(4), 2022, pp. 2151-2180. 10.3150/21-BEJ1413 (arXiv:2011.08321).
2021
Marcin Mider, Moritz Schauer, Frank van der Meulen: Continuous-discrete smoothing of diffusions. Electronic Journal of Statistics 15 (2), pp. 4295–4342, 2021. 10.1214/21-EJS1894, (arxiv:1712.03807).
Joris Bierkens, Sebastiano Grazzi, Frank van der Meulen, Moritz Schauer: A piecewise deterministic Monte Carlo method for diffusion bridges. Statistics and Computing 31, 2021, 10.1007/s11222-021-10008-8, (arxiv:2001.05889).
2020
Joris Bierkens, Frank van der Meulen, Moritz Schauer: Simulation of elliptic and hypo-elliptic conditional diffusions. Advances in Applied Probability 52, pp. 173–212, 2020, 10.1017/apr.2019.54.
Sebastiano Grazzi, Marcin Mider, Frank van der Meulen, Moritz Schauer: Bayesian inference for SDE models: a case study for an excitable stochastic-dynamical model. https://nextjournal.com/Lobatto/FitzHugh-Nagumo, Project: Nextjournal Scholarship for Explorable Research, 2020.
Frank van der Meulen, Shota Gugushvili, Moritz Schauer, Peter Spreij: Nonparametric Bayesian estimation of a Hölder continuous diffusion coefficient.Brazilian Journal of Probability and Statistics 34 (3), pp. 537–579, 2020, 10.1214/19-BJPS433. (pdf)
2019
Frank van der Meulen, Shota Gugushvili, Moritz Schauer, Peter Spreij: Fast and scalable non-parametric Bayesian inference for Poisson point processes. RESEARCHERS.ONE, 2019, https://www.researchers.one/article/2019-06-6, with comments by Paulo Jorge de Andrade Serra, Ryan Martin and Adeline Samson.
Frank van der Meulen, Shota Gugushvili, Moritz Schauer, Peter Spreij: Bayesian wavelet de-noising with the caravan prior. ESAIM: Probability and Statistics 23, pp. 947–978, 2019, 10.1051/ps/2019019.
Denis Belomestny, Shota Gugushvili, Moritz Schauer, Peter Spreij: Nonparametric Bayesian inference for Gamma type Lévy subordinators. Communications in Mathematical Sciences 17 (3), pp. 781–816, 2019, 10.4310/CMS.2019.v17.n3.a8.
Frank van der Meulen, Shota Gugushvili, Moritz Schauer, Peter Spreij: Nonparametric Bayesian volatility estimation. In: David R. Wood et al. (ed.): 2017 MATRIX Annals, Springer, 2019, ISBN 978-3-030-04160-1, 10.1007/978-3-030-04161-8_19.
2018
Ruifei Cui, Perry Groot, Moritz Schauer, Tom Heskes: Learning the causal structure of copula models with latent variables. In: 34th Conference on Uncertainty in Artificial Intelligence 2018, UAI 2018. EID: 2-s2.0-85059432907.
Frank van der Meulen, Moritz Schauer: Bayesian estimation of incompletely observed diffusions. Stochastics 90 (5), 2018, pp. 641–662, 10.1080/17442508.2017.1381097.
Frank van der Meulen, Moritz Schauer, Jan van Waaij: Adaptive nonparametric drift estimation for diffusion processes using Faber-Schauder expansions. Statistical Inference for Stochastic Processes 21 (3), 2018, 10.1007/s11203-017-9163-7.
2017
Frank van der Meulen, Moritz Schauer: Bayesian estimation of discretely observed multi-dimensional diffusion processes using guided proposals. Electronic Journal of Statistics 11 (1), 2017, 10.1214/17-EJS1290.
Moritz Schauer, Frank van der Meulen, Harry van Zanten: Guided proposals for simulating multi-dimensional diffusion bridges. Bernoulli 23 (4A), 2017, pp. 2917–2950, 10.3150/16-BEJ833.
Before 2015
Frank van der Meulen, Moritz Schauer, Harry van Zanten: Reversible jump MCMC for nonparametric drift estimation for diffusion processes. Computational Statistics & Data Analysis 71, 2014, pp. 615–632, ISSN 0167-9473, 10.1016/j.csda.2013.03.002.
Christos Pelekis, Moritz Schauer: Network Coloring and Colored Coin Games. In: S. Alpern, R. Fokkink et al. (ed.): Search Theory: A Game Theoretic Perspective. Springer, 2013. ISBN-13: 978-146146824, 10.1007/978-1-4614-6825-7_4. Note: The proof therein is based on a uniform bound on the median of the number of sources (resp. sinks) in a graph with randomly oriented edges (randomly oriented graphs) of independent interest.
Selected software publications
Moritz Schauer et al.:Bridge. Zenodo, 10.5281/zenodo.891230. A statistical toolbox for diffusion processes.
Shota Gugushvili, Moritz Schauer: MicrostructureNoise 0.10. Zenodo, 10.5281/zenodo.1241010. 2018. Bayesian volatility estimation in presence of market microstructure noise.
Moritz Schauer: CausalInference 0.4. Zenodo, 10.5281/zenodo.1005091. Julia package for causal inference, graphical models and structure learning with the PC algorithm.
Bibliography and author information
arXiv https://arxiv.org/a/0000-0003-3310-7915.html
http://orcid.org/0000-0003-3310-7915
Preferred names in citations are “Moritz Schauer” and “M. Schauer”. IPA: [ˈmoː/r/ɪts ˈʃaʊ̯ɐ].
Cite work from arXiv (.bib). Download .bib-file with information from doi.org .
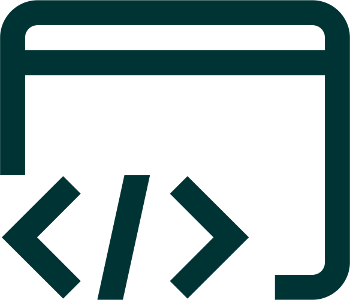
Open source contributions
See github.com/mschauer.
Supervision
Students
Ruben Seyer, supervision.
Marc Corstanje, co-supervision.
Oskar Eklund, co-supervision.
Sebastiano Grazzi (TU Delft), ipso facto.
Google Summer of Code
Frank Schäfer: High weak order solvers and adjoint sensitivity analysis for stochastic differential equations. In: GSoC 2020 (Julialang). Report.
Frank Schäfer: Neural Hybrid Differential Equations and Adjoint Sensitivity Analysis. In: GSoC 2021 (Numfocus). Report.
Archana R Warrier: Causal and counterfactual methods for fairness in machine learning. In: GSoC 2021 (JuliaLang). Report.
Master theses
Hanna Skytt: Change point detection in financial time series in connection to purchase behaviours. 2022. In cooperation with https://www.svalna.se.
Louise Hultén: Causal effect of carbon footprint calculators. 2022. In cooperation with https://www.svalna.se.
Vincent Molin: Bayesian inverse problems with neural generative priors. 2022. With Axel Ringh.
Siddhant Som, Swaathy Sambath: Scene Change Detection. 2022. https://hdl.handle.net/20.500.12380/304951. In cooperation with CEVT.
Noa Onoszko, Gustav Karlsson: PENS: Leveraging Data Heterogeneity in Federated Learning. 2021. In cooperation with: Edvin Listo Zec, RISE.
Krister Ekström: Multivariate linear regression of LIBS spectra. https://hdl.handle.net/20.500.12380/302134, 2020. In cooperation with https://www.swerim.se.
Eva Hegnar: Probabilistic deep learning with variational inference. https://hdl.handle.net/20.500.12380/301602, 2020. In cooperation with https://www.solutionseeker.no.
Erik Hermansson: Latent State Estimation of Financial Time Series: estimating financial health with MCMC methods and particle filters. 2020.
Journal
- 2021/10/11 Nonparametric Bayes and Fourier methods in Julia (Intro Julia/Pluto notebook)
- 2021/07/30 ZigZagBoomerang.jl - parallel inference and variable selection (JuliaCon 2021 | Youtube)
- 2021/06/16 Sensitivity Analysis of Hybrid Differential Equations (with Frank Schäfer)
- 2018/05/17 MicrostructureNoise
- 2018/05/09 Talk: Continuous-discrete smoothing of diffusions (Mannheim Probability and Statistics Seminar)
- 2018/05/08 Talk: Nonparametric Bayesian volatility estimation (Statistik-Kolloquium der Universitäten Gießen und Marburg)
- 2018/01/31 Multivariate stochastic differential equations with Bridge
- 2018/01/19 Parameter inference for a simple SIR model